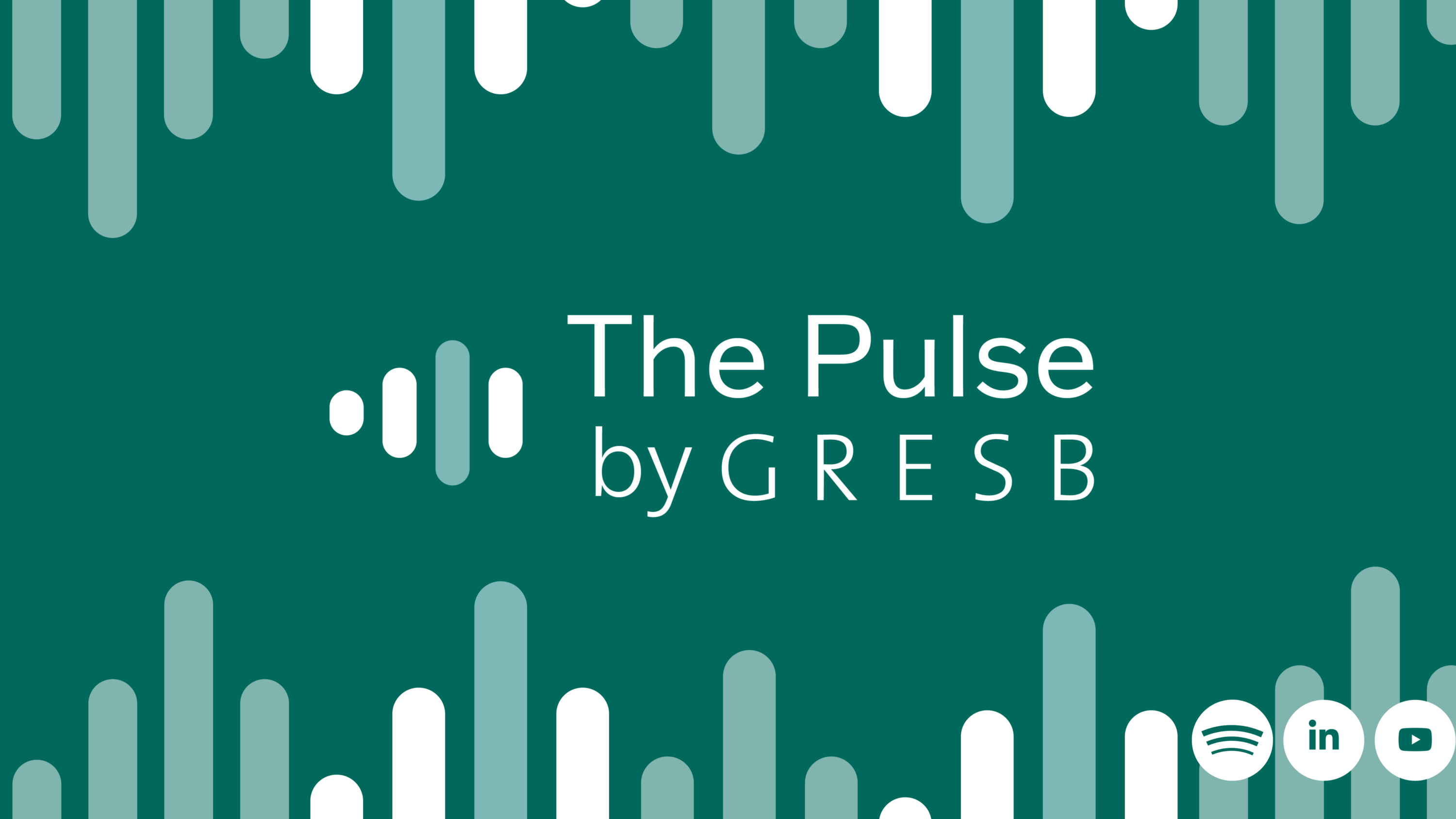
Introducing The Pulse by GRESB
The Pulse by GRESB is a new content series featuring the GRESB team, partners, GRESB Foundation members, and other experts. Each episode features a host from GRESB and at least one interviewee, focusing on an important topic related to either GRESB, ESG issues within real assets industry, decarbonization efforts, or the wider market.
Diving into GEM: The methodology behind REAL
In this episode of The Pulse by GRESB, we bring you an in-depth conversation about GRESB’s estimation model used for REAL Solutions. Our experts begin by detailing the specifics behind the GEM methodology and explore how it aligns with global standards such as the PCAF scoring model. Watch the episode below.
Transcript
Can’t listen? Read the full transcript below. Please note that edits have been made for readability.
Parag: Hello and welcome to The Pulse by GRESB, our new series where we discuss the newest and most topical issues in sustainable real assets, from GRESB and ESG to the wider industry. So today I’m your host, Parag Cameron Rastogi, and we’re going to be talking about the GRESB Estimation Model for asset level data, fondly known as GEM.
And to help me talk about this gem of a model, I’m joined today by Emma Storm, Principal Data Scientist at GRESB, and an all around expert in all things to do with data and machine learning. Hi, Emma.
Emma: Hi, Parag. Thanks for having me here.
Parag: So we’re going to start without any easy questions, we’re going to start with the hardest one. Could you begin by describing to us, what is the GRESB estimation model, or GEM?
Emma: Yeah, so, the GRESB estimation model, or as we like to say, the GEM, our little gem, it fills in missing gaps in performance data, namely energy and GHG data for real estate assets, for buildings. And yeah, it’s a well known problem for managers in the GRESB universe that getting access to data can be quite challenging for some of the buildings that they manage. So the GEM takes advantage of the fact that we do have good coverage across lots of the GRESB universe, and we can help managers fill in missing data by estimating energy use and calculating GHG emissions for their buildings.
Parag: So, if the GEM is an estimation model, it obviously uses some underlying methodology. Could you talk a little bit about this methodology and if GEM has some kind of superpower that would make people want to trust it more and make it more accurate?
Emma: Yeah, so the GEM, I would call it primarily a statistical based model. So, it uses both a combination of an individual asset’s characteristics and whatever energy data this asset has, and it uses this in combination with statistics from benchmark groups to estimate the missing energy data. It’s kind of a combination of extrapolation of existing data and imputation of basically median values from benchmark groups.
Since it uses both an individual asset’s data and also statistical data, it can provide more accurate and more robust estimations than simply using either only individual asset level data via, like, a linear extrapolation. Or using, let’s say, only market-based statistics.
Parag: Interesting. So it’s actually, in a sense, it’s using knowledge from a given market, so country plus a building type, in addition to knowledge from the asset itself. Is there a way to balance the two? Do you have a particular sort of recipe for balancing the two?
Emma: Yeah, so, it comes down to how much data you actually have on your building, basically. So, if you don’t have any data at all, let’s say, that’s one scenario where you know you have an asset that’s in a particular place and is a particular property type but you don’t know much about it. What we’ll be able to do is identify which benchmark group matches your asset’s characteristics and then we’ll fill in the energy used by taking the median intensity of that benchmark group saying, okay, your asset falls into this category, so we’ll put your energy uses based on your benchmark group entirely. But if you give us some data, then we’ll basically start to take that into account. We also do this at a very granular level.
So if you tell us, oh, I have full data coverage or I know exactly what my energy use is for the common areas or the landlord controlled space in your building, then we’ll only apply the estimation model to the tenant spaces. So we’ll be able to fill in your tenant space data for you.
If you know all of the energy use for electricity, but you don’t know anything about the fuel use of your building, then we will also take that into account when estimating the remaining energy. And then basically the idea is that if you have a low data coverage, then extrapolation of a very small amount of data to a whole building is probably not going to result in a very accurate value. But if you have a lot of data coverage, meaning , maybe you’re just missing a few small spaces like one tenant is missing from your office building and you don’t know the coverage for that building, or whatever. Then okay, well, the energy use of that space is probably more similar to the energy use of the rest of the building. So it depends on the data that you give us.
Parag: Yeah, so in a sense what you’re saying is the more data you know from an actual building, the more you use that data to estimate the rest of the missing bits. And the less you know, the more you use sort of market characteristics.
Emma: Yeah exactly.
Parag: Which makes a ton of sense because that is one of the underlying limitations of using models that are either overly reliant on the market, or overly reliant on the partial data provided by the asset itself is in either cases, like you said, if you have very little data from an asset and you extrapolate everything else, it could be wildly biased. So that is an interesting point. And does that mean that the GEM method does better than comparable methods or, assuming the same level of information, of course, if you had perfect information about an asset, you would extrapolate whatever you wanted, but given that we don’t live in a perfect world, did you compare GEM to other methods, and how did it do?
Emma: Yeah, so, we looked at the comparison, of the GEM against simple linear extrapolation, and generally the GEM does quite a bit better at estimating the actual true energy use of the building than linear extrapolation. Especially the lower the data coverage or the smaller the fraction of the building that you have energy data for, the better that the GEM performs relative to linear extrapolation.
Parag: I want to, I want to probe a little bit further into this word that you use, simple, right? Of course, usually we want the simplest model that does the job. That’s sort of a, almost a rule of thumb in machine learning. You should not multiply entities beyond necessary, right?
Occam’s razor. In this case, does the fact that the GRESB model is actually fairly simple, but it uses the power of the GRESB database, was that deliberate choice to ensure that the data is reliable and consistent and robust or is this dictated by what we have?
Emma: Oh no, I think it was a deliberate choice. You know, for a few different reasons, right? Whenever you’re building something new, you don’t want to make it too complicated at the beginning.
We also definitely wanted to have a model that we could make very simple, very easy comparisons with other approaches. And we wanted it to also be easy to explain, and kind of use characteristics that we already know are very important when it comes to understanding the energy use of a building.
So, for the benchmark groups, for example, we use primarily location and property type to determine benchmark groups, right? And so we know that those are the two dominant characteristics in determining energy use. So there’s lots of other things that we could include and, I mean, I think that it would be really fun to explore those, but, as a first initial model, yeah, you kind of want to go with what’s simplest.
Parag: Yeah, yeah, it makes a ton of sense of course and you spoke a little bit about the context within which we are working, and the GEM method at the end of the day does a few different things. It does provide an estimate like on the fly for the user. So you can benchmark your building even if you have partial data against complete buildings, which of course its main use is. But we do of course exist in a market. We exist in the real world and we have to test our assumptions and our methods against the real world.
How does the GEM methodology align to other market forces out there, like PCAF, if we do at all?
Emma: So, PCAF is a framework for carbon accounting for real estate in particular, they have a data quality score that you can use to basically evaluate how good your estimation model is, or rather how good your carbon accounting is.
So, I’ve been talking mostly about the energy estimation part of the GEM, but the other important part of the GEM is that we take this complete picture of your energy use and then calculate your GHG emissions, from that energy data using our own database of location based emissions factors.
So then, depending on what level of energy data that you provide, we can assign a PCAF score to the calculated GHG emissions. And if you have complete energy coverage, that would correspond to option 1B for the estimation approach for PCAF, or a PCAF score of 2. This is because we’re using location based as opposed to supplier specific emissions factors in the GEM.
If you have partial or no energy data coverage, then that would correspond to option 2B or a PCAF score of 4, since we use the location, the asset type, and the floor area all as part of the energy estimation.
Parag: Well, I would love to continue talking, but that’s about all the time we have for today’s episode of The Pulse. So thank you, Emma, for taking the time to join me and sharing your expertise.
Emma: Yeah, thanks. It was a great conversation to have, Parag.
Parag: Well, if you’ve enjoyed the show, don’t forget to give this content a like, share, and leave a comment. We’d love to hear from you about this and other episodes. So please do get in touch at, [email protected]. I’ve been your host, Parag Cameron Rastogi. See you next time on The Pulse by GRESB.